ModernBERT: A New Era for Encoder-Only Transformers
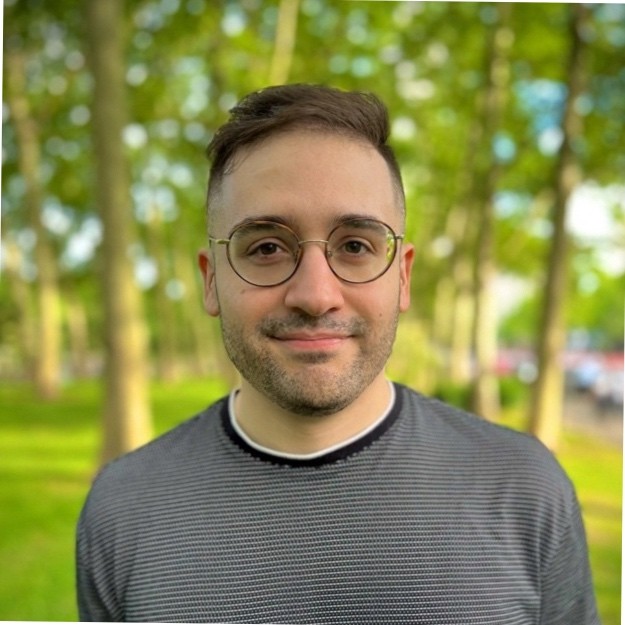

Over the last few years, we have seen tremendous progress in large language models (LLMs). Yet, while decoder-based transformers (like GPT and Llama) have stolen much of the spotlight—particularly for tasks involving text generation and chatbot interfaces—encoder-only models such as BERT (Bidirectional Encoder Representations from Transformers) have continued to power critical applications behind the scenes. Indeed, search engines, text classifiers, recommendation systems, knowledge extraction pipelines, and retrieval-augmented generation (RAG) frameworks all rely heavily on encoders to build high-quality vector representations.
ModernBERT is the new frontier in encoder-only architecture—designed to be smarter, faster, more efficient, and capable of handling longer contexts than its predecessors. In this in-depth blog post, we will walk you through every facet of ModernBERT, from architecture and training procedures to downstream evaluations, to potential use cases and limitations. We’ll also examine how ModernBERT can be used in conjunction with emerging retrieval methods such as GraphRAG, helping you see how the two can coexist or complement each other in the world of large-scale information retrieval and question answering.
Why Encoders Still Matter
While generative models get most of the headlines these days, encoder-only transformers power innumerable tasks that require deep, bi-directional language understanding but not necessarily free-form text generation. These tasks include:
- Semantic search & vector retrieval: Turning documents and queries into high-quality embeddings for dense retrieval or recommendation.
- Classification & NLU: Sentiment analysis, natural language inference, and Named Entity Recognition (NER) frequently rely on strong encoder architectures.
- RAG pipelines: Retrieval-augmented generation, which pairs a generative LLM with a lightweight, high-accuracy encoder to fetch relevant context efficiently.
Historically, BERT has been the gold standard for encoders. But BERT, released in 2018, was limited to 512 tokens and trained on data from a narrower domain. Over the years, the research community identified numerous architectural, training, and efficiency improvements—yet the encoder-only domain had not fully absorbed them. ModernBERT changes this landscape, bringing encoder architectures firmly into the modern era with longer context windows, efficient memory usage, code-awareness, and state-of-the-art performance in tasks spanning classification and retrieval.
From BERT to ModernBERT: Key Upgrades
ModernBERT is described as “Smarter, Better, Faster, Longer”—a reflection of its improvements across all major axes that matter for real-world applications. Let’s unpack its most notable architectural and implementation updates.
Positional Embeddings & Extended Context
Classical BERT relied on absolute positional embeddings and was capped at 512 tokens—sufficient for many short text tasks, but woefully inadequate for some enterprise use cases involving legal documents, scientific papers, or aggregated text from multiple web sources.
ModernBERT introduces:
- Rotary Positional Embeddings (RoPE): These embeddings let the model handle sequence lengths of up to 8192 tokens (and beyond). RoPE also scales more gracefully as context grows, enabling ModernBERT to process longer documents with less performance degradation than older encoders.
- Two-phase training for context extension: ModernBERT first trains at 1024 token length, then systematically extends to 8192 tokens. This ensures it develops robust short-context abilities before fully tackling large-scale context windows.
Local-Global Attention
A standout feature of ModernBERT is its alternating local and global attention mechanism:
- Global Attention Layers (every third layer): Provide full attention over all tokens, capturing complete contextual awareness across a large input.
- Local Attention Layers (other layers): Restrict attention to a sliding window of 128 tokens, greatly reducing computational overhead for those layers without sacrificing local coherence.
By reducing the frequency of global attention, ModernBERT achieves significantly faster inference speeds—especially on large or highly padded inputs—while still encoding broad context effectively.
Gated Linear Units (GLU)
ModernBERT swaps out BERT’s GELU activation for GeGLU, a variation of Gated Linear Units (GLUs). This change:
- Improves parameter efficiency and downstream performance.
- Plays nicely with large-scale training, often leading to more stable optimization.
GLUs have proven beneficial in many large language models, but only recently have they been adopted in encoder-centric designs at scale.
Unpadding & FlashAttention
ModernBERT leverages:
- Unpadding: Often, large input batches contain considerable padding. By removing (unpadded) and concatenating sequences before the embedding layer, ModernBERT avoids wasted computation on empty tokens. This can yield a 10-20% performance improvement.
- FlashAttention: A high-performance kernel that reduces memory usage and speeds up attention operations.
- FlashAttention 2 for local attention.
- FlashAttention 3 for global attention on compatible GPUs (e.g., NVIDIA H100).
By combining unpadding with FlashAttention, ModernBERT can handle large batches at high speeds on both consumer and enterprise-grade GPUs.
Hardware-Aware Design
In the LLM space, multiple studies have shown the importance of matching model dimensions—like hidden size and layer depth—to GPU memory and compute patterns for optimal performance. ModernBERT carefully balances depth vs. width:
- The Base model has 22 layers, hidden size of 768, and an expansion size of ~2,304 in the feed-forward blocks (GeGLU).
- The Large model uses 28 layers, hidden size of 1,024, and feed-forward expansions of ~5,248.
Despite having more parameters than older base models, ModernBERT often outperforms them in speed because it’s tiled effectively for GPU architectures (NVIDIA T4, RTX 3090, RTX 4090, A100, etc.). This means more streaming multiprocessors (SMs) are used efficiently, boosting throughput across short and long contexts.
Training at Scale: Data, Tokenizer, and Schedules
Massive Pretraining Corpus
A major difference between ModernBERT and older encoders:
- Trained on 2 trillion tokens of predominantly English text, including web pages, code, academic articles, and other large corpora.
- Incorporation of code data is especially noteworthy, as it leads to superior performance on tasks like code search and retrieval, without sacrificing general-purpose NLP capabilities.
Improved Tokenization (OLMo-BPE)
Instead of reusing BERT’s original WordPiece tokenizer, ModernBERT deploys an OLMo-based BPE with a 50,368-vocabulary:
- Achieves better subword segmentation, especially in programming languages (braces, punctuation).
- Maintains backward compatibility with BERT’s special tokens like
[CLS]
and[SEP]
.
Optimization & Hyperparameters
ModernBERT employs:
- StableAdamW optimizer: Incorporates gradient clipping strategies from Adafactor for more stable updates.
- High masking rate (30%) for Masked Language Modeling (MLM) to bolster representation richness.
- Learning rate schedule that includes warmup, a stable plateau, and then a 1 – sqrt decay. This approach avoids issues with pure cosine decay and allows smooth restarts.
- Sequence packing: Over 99% packing efficiency ensures minimal wasted tokens in each training batch.
Transition to 8192 Context Length
The model is first trained at 1024 tokens, sees 1.7 trillion tokens, then extends to 8192 tokens with additional 300B tokens. This two-step methodology:
- Preserves short-sequence mastery.
- Successfully scales up to ultra-long contexts.
The result is a model capable of handling tasks that require detailed passage-level understanding, or even entire documents, with minimal performance loss.
Use Cases & Industry Applications
-
Enterprise Semantic Search:
- Ingest tens of thousands of documents (financial reports, knowledge bases) into ModernBERT embeddings; run queries at scale with quick latency.
- Improves user experiences in helpdesks, document management, and corporate search.
-
Retrieval-Augmented Generation (RAG) for Chatbots:
- Use ModernBERT to fetch relevant knowledge while a GPT-like model composes the final response.
- Superior to older BERT-based pipelines due to faster inference and code/data synergy.
-
Code QA and Developer Tools:
- StackOverflow or GitHub-based Q&A.
- Integrated environment assistants searching code repositories more accurately thanks to code training.
-
Recommendation Systems:
- In recommendation engines (e.g., e-commerce, media), vector embeddings capturing item-user or item-item relationships are crucial.
- ModernBERT can handle larger descriptions/reviews thanks to 8k token context.
-
Academic or Legal Document Summarization:
- Even though ModernBERT is not generative, it can cluster or embed full academic papers or legal briefs, enabling robust classification and “find similar docs” tasks.
- Potential synergy with GraphRAG for truly global sensemaking.
Performance Benchmarks
ModernBERT is evaluated on a broad range of tasks that reflect modern enterprise and research needs.
GLUE for Natural Language Understanding
The GLUE benchmark remains a gold standard for classification and NLU:
- ModernBERT-base surpasses older strong encoders like DeBERTaV3-base and RoBERTa-base, achieving new state-of-the-art results in the “base” category.
- ModernBERT-large nearly ties DeBERTaV3-large on average metrics, while being faster at inference and having fewer parameters.
Implication: If your use case is strictly NLU, ModernBERT offers a clear advantage over legacy BERT-based models in both accuracy and speed.
Retrieval (DPR, ColBERT, and Beyond)
ModernBERT’s major design objective is to shine in semantic search and retrieval-augmented generation pipelines.
-
Dense Passage Retrieval (DPR)
- Trained on MS MARCO with contrastive learning.
- On the BEIR suite, ModernBERT-base and -large surpass GTE-en-MLM, NomicBERT, RoBERTa, and the original BERT in retrieval scores (nDCG@10).
-
ColBERT (Multi-vector retrieval)
- By representing each token with a separate vector and using MaxSim for document-query scoring, ColBERT reduces loss of contextual detail.
- ModernBERT’s strong long-context capabilities show especially large gains here. For tasks requiring extended passages or multiple paragraphs, ModernBERT-based ColBERT outperforms other encoders by 9–10+ nDCG points.
-
Long-context retrieval
- Evaluated on MLDR, which has documents up to thousands of tokens long.
- ModernBERT consistently leads in multi-vector setups. With single-vector setups, it closes the gap significantly once it is fine-tuned on longer sequences.
Implication: If you’re building a search engine, question-answering system, or RAG pipeline with doc sizes up to 8k tokens, ModernBERT drastically cuts inference time and improves ranking accuracy.
Code-Aware Tasks
ModernBERT is unusual among encoders in that it was explicitly trained on a substantial volume of code:
- On CodeSearchNet (matching code snippets to docstrings) and StackQA (a hybrid text+code retrieval dataset), ModernBERT easily outperforms RoBERTa, DeBERTaV3, and GTE-en-MLM, sometimes by large margins.
- The incorporation of code data does not degrade its performance on standard NLP tasks; in fact, synergy between code and language data often leads to better general reasoning about structure.
Efficiency Metrics & Inference Speed
On an NVIDIA RTX 4090 (a consumer-grade high-end GPU), ModernBERT achieves:
- Short context (512 tokens):
- ModernBERT-base processes 148k tokens/s with minimal memory overhead—faster than many specialized encoders with fewer parameters.
- Long context (8192 tokens):
- ModernBERT processes up to ~46–52k tokens/s, 2-3x faster than next best open-source encoders, thanks to unpadding and local-global attention.
- Memory efficiency:
- Able to handle larger batch sizes than GTE-en-MLM or DeBERTaV3 at both short and long contexts.
- This advantage translates to lower TCO (total cost of ownership) if you’re running large-volume inference in enterprise settings.
Combining ModernBERT & GraphRAG
ModernBERT and GraphRAG address overlapping but ultimately distinct challenges in retrieval-augmented pipelines. By combining them, you can harness ModernBERT’s strength in efficient, high-fidelity embeddings (for local or mid-range queries) and GraphRAG’s structured, global sensemaking approach.
Let's make sure we understand what each component does:
-
ModernBERT
- Encoder-Only Model: Great for creating dense vector representations of text or code segments.
- Performance and Speed: Designed for fast inference at both short and long contexts, making it ideal for high-volume applications (e.g., enterprise semantic search or real-time retrieval).
- Local/Focused Queries: Typically excels at tasks like “Find me the top-k documents relevant to X” or classification-based tasks where the entire question can be answered by localized references.
-
GraphRAG
- Graph-Based Index: Constructs a knowledge graph from the corpus, detects communities of related entities, and pre-summarizes them.
- Global Summarization: Ideal for queries that require high-level coverage across the entire dataset (e.g., “What themes are emerging in these 10,000 documents?”).
- Multi-Document Aggregation: Summaries from multiple “communities” in the graph are combined to form a holistic answer.
Together, ModernBERT and GraphRAG can meet two complementary needs:
- Rapid, precision-driven retrieval for specific queries (ModernBERT).
- Global sensemaking or “big-picture” summarization (GraphRAG).
ModernBERT & GraphRAG Workflow
-
Entity/Chunk Extraction
- Use ModernBERT to segment or embed documents, then quickly detect and extract named entities, relationships, or relevant text chunks.
- These embeddings can help group similar entities or text blocks before the graph-building stage in GraphRAG.
-
Graph Construction
- GraphRAG typically builds a knowledge graph by linking together extracted entities and relationships.
- ModernBERT’s embeddings can be an initial step to cluster or rank relevant text chunks/entities. GraphRAG then uses an LLM (or a set of LLM prompts) to transform those chunks into node and edge descriptors in the graph.
-
Community Detection and Summaries
- GraphRAG partitions the graph into communities of thematically related text.
- Here, ModernBERT might also be used to generate sub-summaries or confirm similarity among nodes, since it can quickly gauge how semantically close two chunks are.
-
Query Processing
- For focused queries—“Is there code snippet or a paragraph specifically referencing X?”—you can rely primarily on ModernBERT embeddings to retrieve top-k results rapidly.
- For broader, “global” queries—“What overall trends do these documents describe about technology regulation?”—GraphRAG’s map-reduce summarization steps come into play. GraphRAG will summarize each community, then combine those partial summaries into a single high-level answer.
-
Answer Generation
- After GraphRAG has generated a broad summary, you can still feed any extracted paragraphs, code snippets, or text that ModernBERT found into a generative model to produce final, user-facing text—particularly if you want fully fluent natural language answers.
Possible Drawbacks to Consider
-
Index Construction Overhead
- Building a graph in GraphRAG requires additional data preprocessing and LLM calls (for summarizing entities/relationships). This can be time-consuming if the dataset is massive and updated frequently.
- ModernBERT alone is simpler to maintain for purely local queries (vector embeddings).
-
Consistency Between Embedding-Based Retrieval and Graph Structure
- If you’re filtering or “pre-trimming” the corpus with ModernBERT embeddings before building the graph, you could accidentally exclude data that might be relevant for global questions. The pipeline design must ensure synergy rather than a disjoint filter.
-
Complexity
- Architecting a pipeline that first uses ModernBERT embeddings, then GraphRAG indexing, then possible LLM generation may be too complex for smaller teams or simpler search needs.
Impact of Combining ModernBERT & GraphRAG
-
Richer Q&A Experience
- Straight-to-the-point answers for user queries (ModernBERT-based retrieval) plus multi-document, multi-theme overviews (GraphRAG) in one unified pipeline.
- This can be especially valuable in customer support, journalism, market research, and legal discovery workflows.
-
Enhanced Human-Like Sensemaking
- GraphRAG’s multi-level community summaries resemble how humans group ideas thematically, and ModernBERT’s encoder provides the robust “muscle” of local-level retrieval.
- Together, they mimic both the fine-grained reading and broad conceptual grouping that humans use to understand large collections of texts.
-
Reusable Index Structures
- The knowledge graph built by GraphRAG can be repeatedly updated or extended, and ModernBERT embeddings remain valid. Once these structures are in place, new user queries can be resolved faster, with less overhead.
When you combine ModernBERT with GraphRAG:
- ModernBERT handles the where to look (finding the right chunks, boosting relevant text blocks, embedding new documents), quickly and accurately.
- GraphRAG handles the how to synthesize (globally summarizing, linking entities across the entire corpus).
This integration offers scalable, high-performance retrieval for specific questions while supporting broad, multifaceted insights for queries that need big-picture sensemaking. The main implication is that systems can now excel at both hyper-local, detail-driven search and global, multi-document summarization—all in a unified pipeline that remains relatively efficient and user-friendly.
Trade-Offs & Limitations
- Monolingual Focus:
- Trained mainly on English (and code). For multi-lingual or cross-lingual tasks, additional training data or specialized “ModernBERT-multilingual” variants would be needed.
- Resource-Intensive Training:
- Requires large GPU clusters and careful, staged training to handle 2 trillion tokens. Not straightforward to replicate from scratch.
- However, the open-source release with all checkpoints significantly reduces re-training necessity.
- MLM-Only Objective:
- ModernBERT outperforms older BERT models, but might see further gains from advanced objectives like Replaced-Token Detection (RTD). This remains an open area for future innovation.
- Non-Generative:
- Encoder-only models can’t produce multi-sentence answers in the same flexible manner as GPT or T5. They are most suitable for embedding, classification, retrieval, or short masked predictions.
Conclusion & Outlook
ModernBERT represents a generational leap in encoder-only transformer architecture. It merges:
- Long context (8k tokens)
- Local-global attention for efficiency
- Up-to-date code and text training data (2 trillion tokens)
- FlashAttention & Unpadding to push performance on consumer and server GPUs
- High retrieval and classification accuracy that sets new benchmarks across GLUE, BEIR, code tasks, and more.
With an Apache 2.0 license and open availability on platforms like Hugging Face, ModernBERT is poised to become the de facto standard for organizations demanding cutting-edge retrieval and text understanding solutions. It aligns well with advanced RAG frameworks, can be combined with GraphRAG for global summarization tasks, and integrates naturally into any AI pipeline that benefits from rapid embedding generation.
Looking Ahead: Expect expansions into multilingual variants, potential synergy with advanced objectives (like RTD), and continued hardware optimizations. ModernBERT underscores a simple truth: Encoder-only transformers remain crucial to the evolving landscape of AI, offering specialized and highly efficient solutions for knowledge-based tasks, retrieval-augmented generation, and the next wave of intelligent agent frameworks.
Further Reading & Resources
- ModernBERT GitHub: github.com/AnswerDotAI/ModernBERT
- ModernBert Paper: Warner et al. (19 Dec 2024) Smarter, Better, Faster, Longer: A Modern Bidirectional Encoder for Fast, Memory Efficient, and Long Context Finetuning and Inference
- GraphRAG Paper: Edge et al. (24 Apr 2024). From Local to Global: A Graph RAG Approach to Query-Focused Summarization